Graphs, Embeddings, and LLM-Generated Jokes
2025-04-11I started Recurse Center last week as a part of the Spring 2 2025 batch and am having a blast so far. This was my first mini project of the batch.
Ever since GPT-2 was announced, I’ve been curious whether or not LLMs can be funny. Somewhat surprisingly, the latest reasoning models are outclassing the majority of humans at mathematical problem solving, but at least anecdotally, they struggle with writing jokes and assessing humor. My guess is that this has to do with how challenging it is to quantify humor in the first place, making training for “funniness” a difficult thing to benchmark, let alone compute gradients for.
In this post, I talk about how I apply a theory of joke construction as a framework for LLM-generated humor. The approach takes us through some very light graph theory, text embeddings, and of course LLM prompting. In the end, the jokes are, dare I say, even a little bit funny.
A structure for jokes
A few months ago, I came across this article describing a theory and structure behind jokes. It doesn’t try to explain all of humor, but it provides a solid groundwork from which to write short one- or two-line lines jokes. I found the article fascinating, and was immediately curious if LLMs could leverage the ideas it presents.
The core argument of the post is that humor relies on subverting expectations. This can be restated as taking two unrelated subjects (the setup and the punchline) and joining them with some common aspect. This way, the setup and common aspect work together to simultaneously set expectations, and also break them (to a reasonable degree) using the punchline.
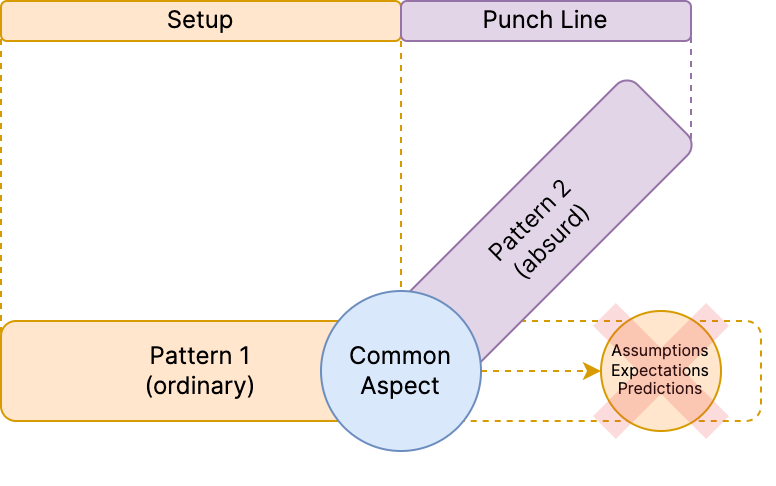
This is best shown with an example:

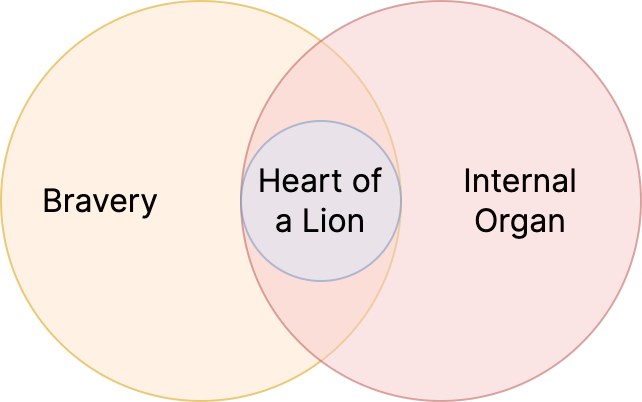
This is clearly a promising way to start generating jokes. To begin, we need to start generating these “triplets” of setup, common aspect, and punchline.
Generating setups, punchlines, and common aspects
The obvious way generate these triplets automatically would be ask to an LLM. Unfortunately, creativity here isn’t their strong suit:
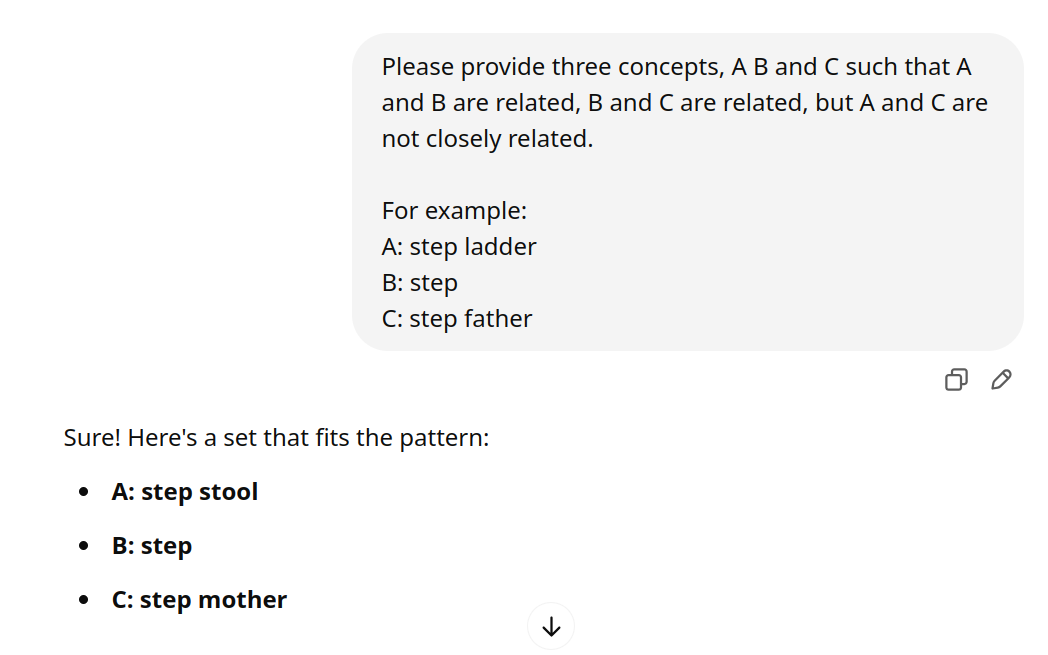
Perhaps unsurprisingly, LLMs seem to struggle with both randomness and unexpected connections between topics. Instead, we can simplify the task and only ask them to find adjacent or similar topics, and then navigate the graph that’s formed to find unexpected connections:
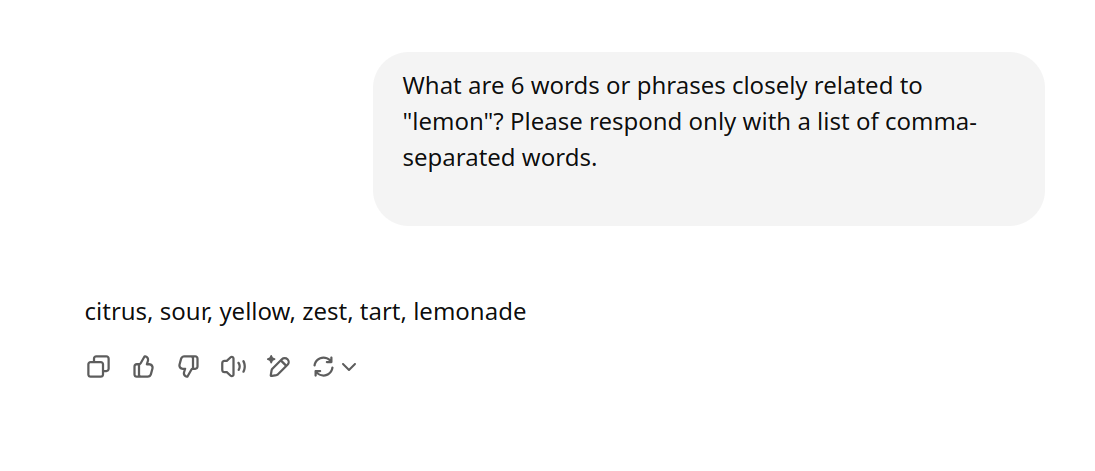
Using this prompt, we can run a breadth-first-search by repeatedly asking our LLM to find more adjacent topics. This requires just a few tokens per query and is surprisingly inexpensive even using the large (non-reasoning) frontier models.
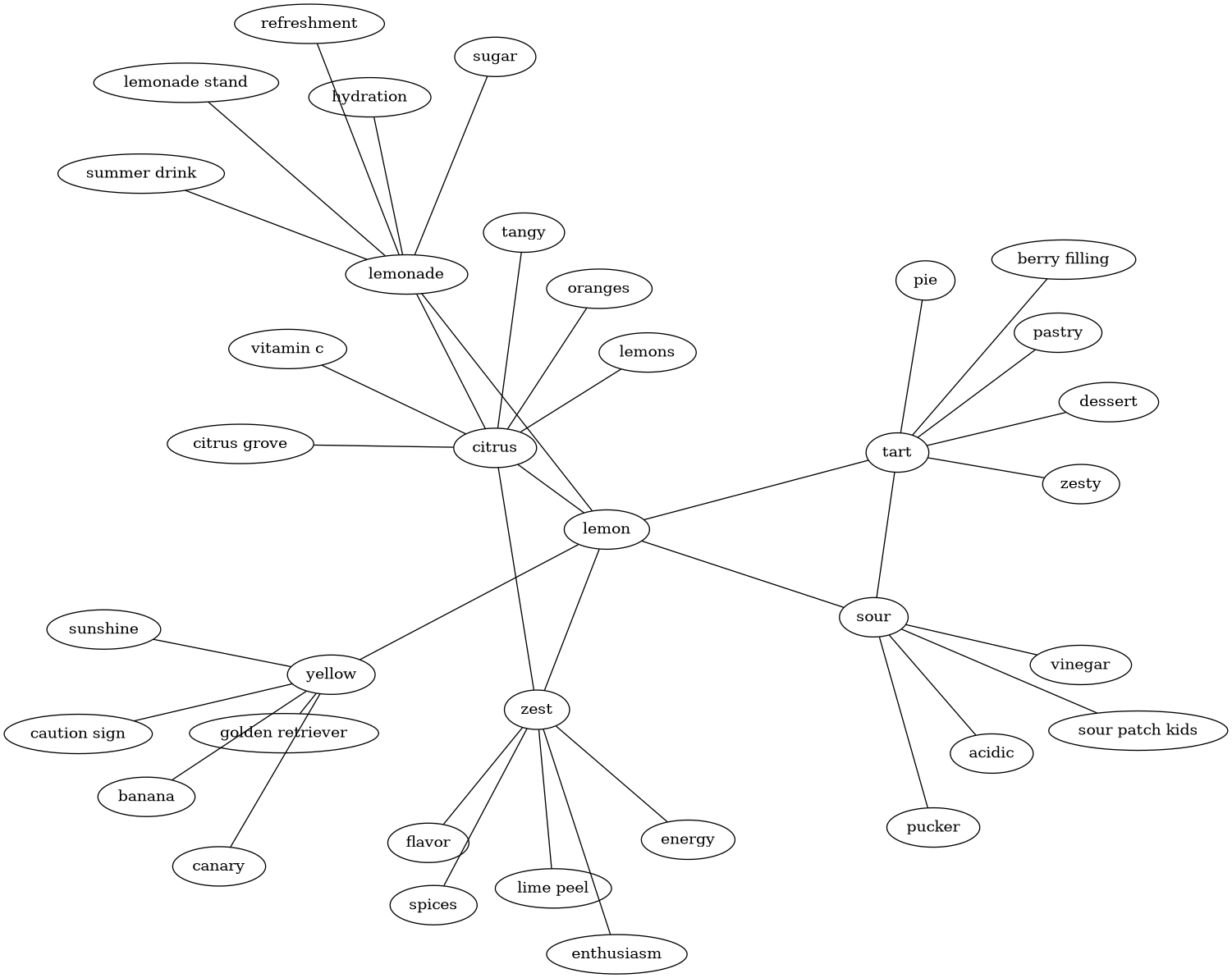
Finding unrelated setups and punchlines
Now that we have a graph of concepts, we can pick a random node, and look at the 2nd-degree neighbors to generate these setup, common aspect, and punchline triplets. However, without further guidance, this approach will often produce triplets like (“lemon”, “citrus”, “lime”), which clearly fails to subvert expectations. A triplet like (“lemon”, “yellow”, “golden retriever”) is a much stronger triplet. But how do we quantify that?
Luckily, this is where text-embeddings shine. Text-embeddings convert text into high-dimensional vectors, which unlike text, can easily be compared numerically. They are typically used to rank similarity between passages of text, augmenting search algorithms with the ability to find similar “concepts” rather than just matching keywords. In our case, we can search to minimize the vector similarity between setups and punchlines, relying on our graph to provide candidates linked by a common aspect.
Using our earlier example and cosine similarity yields:
dot(lemon, lime) = 0.8718...
dot(lemon, golden retriever) = 0.2144...
The jokes
Now that we can generate a setup, common aspect, and punchline, all that’s left is to provide them to an LLM and explain the joke writing structure. Doing this, I generated nearly one thousand jokes. Maybe ~10% were kind of funny. Here are some of my favorites:
-
My nutritionist told me the secret to a balanced diet is burning exactly as many calories as you eat. I think I’ve mastered counting them, but lighting spaghetti on fire is harder than you think.Setup: balanced diet, Common Aspect: calories, Punchline: burning
-
Puberty was tough before social media – back then, teenagers had to go door-to-door to find out nobody liked them.Setup: puberty, Common Aspect: teenager, Punchline: social media
-
I accidentally ran
git prune
on my backyard, now I’m left with nothing but twigs.Setup: version control, Common Aspect: branches, Punchline: twigs -
My therapist told me delayed gratification was the secret to happiness. I’m now perfectly content – still waiting for his next appointment slot to open up.Setup: delayed, Common Aspect: gratification, Punchline: contentment
-
My family asked to preserve my grandfather’s legacy. Turns out, army medals don’t keep well in pickle jars.Setup: legacy, Common Aspect: preserving, Punchline: pickling
-
I took the subway today. It was crowded, uncomfortable, and honestly, tasted nothing like advertised.Setup: public transit, Common Aspect: subway, Punchline: sandwich
-
I tried to update my computer, but it was still thirsty – it just kept asking me to refresh.Setup: update, Common Aspect: refresh, Punchline: thirst
-
My banker asked if I was satisfied with my interest. Turns out she didn’t really care about my model train collection.Setup: banks, Common Aspect: interest, Punchline: fascination
-
My therapist told me I needed more chill in my life, so I bought twenty pounds of frozen yogurt – I’m still anxious, but now it’s mostly about freezer space.Setup: frozen yogurt, Common Aspect: chill, Punchline: relax
-
My utility bill was so shocking this month, I’m wearing rubber gloves to open next month’s.Setup: utilities, Common Aspect: electricity, Punchline: shock
-
I’ve always believed my emotional inner world was private and mysterious – unfortunately, nobody explained that to my eyebrows.Setup: inner world, Common Aspect: emotions, Punchline: expression